Swiss cheese and spice: McMaster lab combats antibiotic resistance with AI
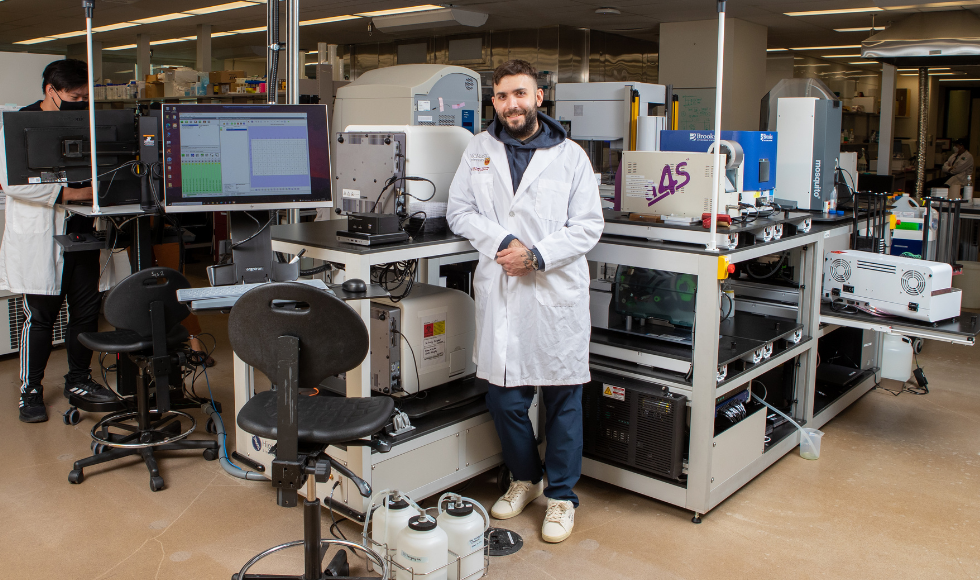
Jon Stokes, an assistant professor of biochemistry & biomedical sciences, is using artificial intelligence and machine learning to explore new options to combat antibiotic resistance. (Photo by Georgia Kirkos/ McMaster University)
BY Jesse Dorey
February 24, 2023
Antibiotic resistance is rapidly becoming a pandemic-sized problem.
“Bacteria are evolving ways to overcome existing antibiotics faster than we can invent new ones,” says Jon Stokes, assistant professor of biochemistry and biomedical sciences. “As a result, we end up seeing more and more patients with drug-resistant bacterial infections that we can’t treat, which significantly increases illness and death.”
It’s a problem that could have been avoided, says Stokes.
Enter artificial intelligence (AI). Stokes and his research team are using AI and machine learning to explore new options to combat antibiotic resistance.
And not a moment too soon. Roughly 4.95 million people died of causes associated with antibiotic resistance in 2019. By 2050, it’s estimated this number will approach 10 million per year.
This is because bacteria, thanks in part to overuse in agriculture and medicine, are finding ways to outsmart the antibiotics we’ve relied on for nearly a century to treat common bacterial infections, such as strep throat.
Additionally, a wide array of routine medical procedures heavily rely on the use of antibiotics, further complicating an already dire situation.
But it takes a tremendous amount of time and resources to develop new antibiotics using the two traditional forms of discovery – natural product discovery and synthetically produced small molecules.
That’s what led Stokes and his research team to take a novel approach. It’s time to use smart tech to outsmart bacteria.
CONSIDER THE SWISS CHEESE
In an academic setting, a lab using traditional methods of antibiotic discovery will be capable of screening thousands to tens of thousands of molecules – maybe even a few hundred thousand at the very largest of scales.
While that number seems large, there’s an astronomical number of potential drug-like chemicals that could theoretically exist – we’re talking on a scale larger than the approximate number of atoms that make up our entire solar system. This means that the scale of these laboratory tests are small peanuts compared to the theoretical limit.
For Stokes’ team, that’s precisely why AI shows so much promise.
“Through the application of AI and machine learning specifically,” explains Gary Liu, graduate student and emerging machine learning expert in Stokes’ lab, “we can rapidly find and explore untapped chemical spaces where we can potentially find new and clinically useful antibiotics.”
In other words, the use of machine learning algorithms provides researchers with the ability to explore far more possibilities than ever before in lab settings.
To understand this process in simpler terms, Stokes paints a vivid picture by comparing the process to, of all things, Swiss cheese.
As we know, Swiss cheese has holes in it.
If you take any number of slices of Swiss cheese and stack them up, one on top of the other, those holes don’t always overlap. But there will inevitably be places where some of them do, allowing you to see right through the pile.
Those places where the holes line up are precisely what the algorithms are searching for and finding.
“We’re finding that tiny little piece of chemical space where we have a molecule that satisfies all the properties that we want it to satisfy, such as strong antibacterial activity, non-toxicity to people and good stability in the complex environment of the human body,” says Stokes. “And we can see through that giant stack of Swiss cheese.”
This process can screen millions – even billions – of different molecules with the push of a button (or many buttons, to be more precise).
THE SPICE MUST FLOW
What makes Stokes’ lab a one-of-a-kind operation isn’t the fact that their models can screen so many molecules in a day, but rather their ability to transition seamlessly from the computer to the lab bench and back again.
While machine learning opens countless doors for the team, they recognize that these models aren’t perfect. This is why, for Stokes’ team, the importance of that human perspective cannot be overstated.
And that’s where his team likes to say they add a little bit of spice – the human perspective and expertise each of them brings – to machine learning.
Here’s what the process looks like in practice:
Working with a machine learning model Liu had previously developed to predict new antibacterial molecules, Denise Catacutan, a graduate student, and an emerging leader in wet lab exploration of new antibiotics, tested these molecules against a collection of bacteria in the lab.
The molecules were not quite as potent as the model suggested.
Instead of being satisfied with these results, though, Catacutan started combining these molecules with other chemicals that allow them to perform better once inside bacterial cells.
Suddenly, many of these molecules worked with high potency against bacteria, exactly the way Liu’s model had predicted.
“That’s where your human eye comes in,” says Catacutan. “Maybe the models are correct, but we just need to add a little bit of spice to really give these molecules that extra push to make them ideal in the lab.”
Because of Catacutan’s work, the team can now take that new data and feed it back into their model to re-train it and make it smarter. Without that hands-on experimentation, none of that model re-training would be possible.
“I like to think that these algorithms are making reasonable suggestions so we can make better decisions,” says Stokes. “This is an example of the marriage between the capabilities and limitations of AI, and the importance of true biological wet lab expertise.”
THE PERFECT MARRIAGE
With the perfect combination of machine learning expertise and hands-on knowledge, the team is hopeful that they can continue to refine their models and improve their results.
According to Telmah Lluka, graduate student under the co-supervision of Stokes and McMaster researcher Eric Brown, and an emerging artificial intelligence expert, the potential that AI shows goes far beyond initial chemical discovery.
You can begin to apply AI at every step of the antibiotic development process, Lluka says.
“How does your new antibacterial compound work? Is it a toxic compound? Can it be used in vivo? You can apply [AI] to pretty much every phase of that pipeline, given that you have robust data to train these models with.”
For Stokes and his team, AI presents a cost-effective and time-effective solution with a world of potential, when used appropriately.
And, when used in combination with that trademark spice of theirs, it has the potential to revitalize the entire antibiotic discovery pipeline.